This comic hits home because it plays out in so many situations:
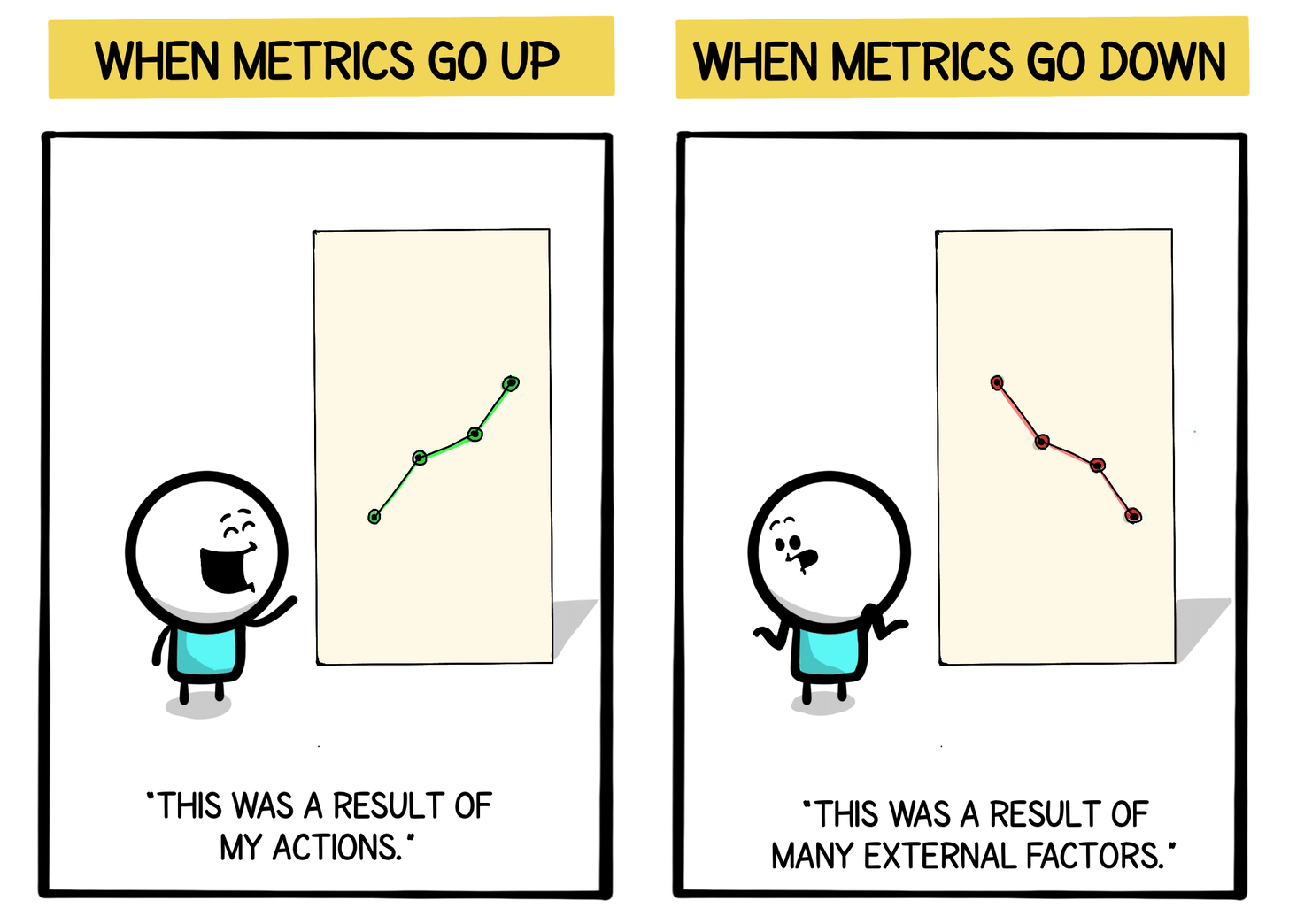
When it comes to metric analysis / data analysis, there are three levels of thinking:
- Level 1 thinking: stick to comfortable takeaways that fit the narrative
- Level 2 thinking: take wild guesses on why metrics are up or down
- Level 3 thinking: analyze the why behind the metrics
Level 3 thinking is rare because it goes against your natural impulses. But this analytical skill is extremely valuable because it helps you reliably get to the root cause, and uncover hidden insights. The best part is that everyone can get there with some guidance, even if you’re not naturally a “numbers person”.
The aha moment that unlocks Level 3: seeing metrics as part of a connected system that reflects how a product works. There is a business equation that describes the whole system. The best way to analyze metrics is to develop a disciplined approach to finding that equation.
Let’s see this in action with a hypothetical scenario. This is a common data crisis at work, and often used in analytical interviews.
Substack comments drop by 25%
Let’s pretend you work at Substack, and noticed that comments on articles have dropped by 25%! It’s your first week, and you are nervous. How do you investigate?
- Importance: does this metric matter?
- Time frame: over what time frame did this metric change?
- Variance: is this fluctuation normal?
- Equation: what is the equation leading to this metric?
- Lever: what is the specific lever that changed?
Steps 1, 2 and 3 are about determining whether you need to be concerned at all. Steps 4 and 5 dive deeper to identify the root cause once you’ve validated it’s worth your time.
Do you need to be concerned?
Step 1 is about importance, and checks whether this metric matters for the business. To answer this, you should return to the mission.
Why does Substack exist? To make it easier for independent writers to build a direct relationship with readers through an email newsletter. While comments is one indication this is happening, the more important metrics should directly measure progress towards its mission, including: # active creators, # free / paid readers, # views on a weekly or monthly basis.
In short, comments are not a tier-1 metric (phew!), but given a 25% drop sounds big, let’s dig a little further.
Step 2 is about time frame: is the 25% drop in comments over the last hour, day, week, month? Metrics fluctuate more in a shorter time frame (e.g., a big writer can draw a lot of comments, which makes an hour or a day later look bad in comparison). You find that it was over the last month, and it does not look like the previous month was inflated by specific writers. The plot thickens.
Step 3 is about variance: is the 25% monthly drop a normal phenomenon? Has it happened before? You find that the biggest monthly drop up until now was 5% over a year ago, so this is definitely a standalone event.
To recap, you know it’s not a tier-1 emergency, but the monthly drop is big enough that it’s worth further investigation.
What’s the root cause?
Step 4 is about finding the equation to help solve your mystery. What is the equation leading up to # comments? Consider what needs to happen for a comment to appear: writers have to publish articles that generate views, some of which lead to comments.
# comments = # published articles * # views/published article * # comments/view
The remarkable benefit of having an equation is that you have a comprehensive, high-level map of where to investigate. You are no longer lost in the forest.
Step 5 is about levers: what is the specific lever in your equation that has changed? Over the last month, # comments dropped by 25%, which means at least one of the three levers in your equation changed. You find that views per article and comments per view were steady, but # published articles dropped by around 25%. This checks out. If you have fewer published articles, there is less content to comment on. But why did # published articles drop?
Once you’ve isolated a lever, you can return to Step 4 to further decompose an equation. Consider what needs to happen for an article to be published: a writer goes on Substack, starts a draft, then hits publish.
# published articles = # active writers * # drafts/writer * # published articles/draft
You find that over the last month, most of the drop came from the second lever, # draft articles made per writer. Very curious. This means writers active on Substack drafted fewer articles this month compared to last month.
You dig into the shift in activity among active writers, and find that a recently launched feature called “Find Writers I Follow” has been so popular that it’s taken activity away from the Drafts section!
You’ve isolated a drop in monthly comments to a drop in published articles, and traced it back to fewer drafts per writer as a result of a new flashy feature taking their attention. Human behavior is fascinating, and you’re now able to observe it at scale.
To make this metric / data analysis faster in the future, you can automate Step 4 which identifies the business equation. By setting up dashboards that expose key levers of the equation, you can quickly spot culprits and eliminate unnecessary fire alarms.
This 5-step framework can be applied to solve any data mystery, from an explosion in Reddit comments, to a decline of Instagram likes, and stagnating marketplace GMV. It’s a lifesaver when metrics are down, but also a useful check when metrics are up.
Recap
Turquoise Boy loves to take credit and celebrate when metrics are up, but just as there’s an underlying story behind why metrics are down, there’s a similar one for the reverse. You should always be able to find the source of growth, decline or stagnation. This helps you reliably distill signal from noise.
I find there’s an unproductive narrative that you’re either a “numbers person” or you’re not. Nobody is born with the ability to analyze data. It comes from exposure, curiosity and practice. You also don’t need to know how to write complicated formulas or even queries to be data-fluent. Data fluency starts with asking questions like those in the framework, which anyone can do.
We are living in a tsunami of data. Learning to surf the waves and find the treasure chest of insights gives you a massive edge. It’s never too late to sharpen your analytical skills.
👋 P.S. Check out who's newly funded and hiring or compare your startup salary & equity!