I just had a blast doing deep analysis. While watching the Golden Bachelor, I caught the culprit behind a traffic drop, and came up with a concrete list of things to fix. My dirty little secret? ChatGPT did the heavy-lifting — it sifted through my data, plotted graphs, and made me feel like a superhuman.
Its sheer speed and competence is straight out of a “living in the future” moment. If you get paid to do analysis, this is scary. If you dread doing analysis, this is good news but still a little eerie. If you run an AI business, this is the beginning of the end, or the start of the beginning… depending on what you do.
No matter how you dice it, value has shifted. Let’s talk about what it means.
Data is cheap, now so is analysis
In my early days at a startup, I had no data support. I feel like a dinosaur saying this, but I would often spend 70% of my day as a PM writing SQL or doing some kind of data work to inform what to build and why.
Even as I got data support, every request still carried a high price tag. Someone had to roll up their sleeves and wrangle the numbers each time, over and over.
Using ChatGPT for analysis is a strange feeling because it’s like getting a smart teammate to do unlimited number-crunching… for $20. This is high-quality, technical work for dirt cheap.
Growing up, a common advice was to learn “something technical” — a hard skill that any employer would value. That world has changed. But before we go there, a moment of appreciation for the work that blew my mind.
ChatGPT analysis in action
I started with a hypothesis that either search volume dropped for my keywords, or search ranking slipped, or both:
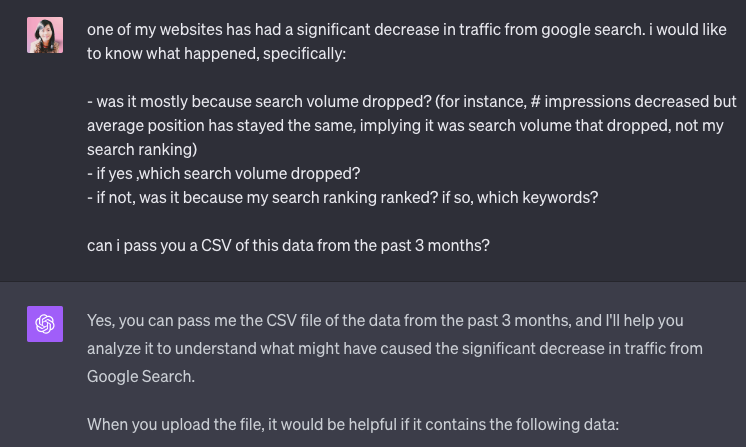
We go back and forth a bit about which files to upload, and then, unprompted, it plots my crazy CSVs into some neat graphs:
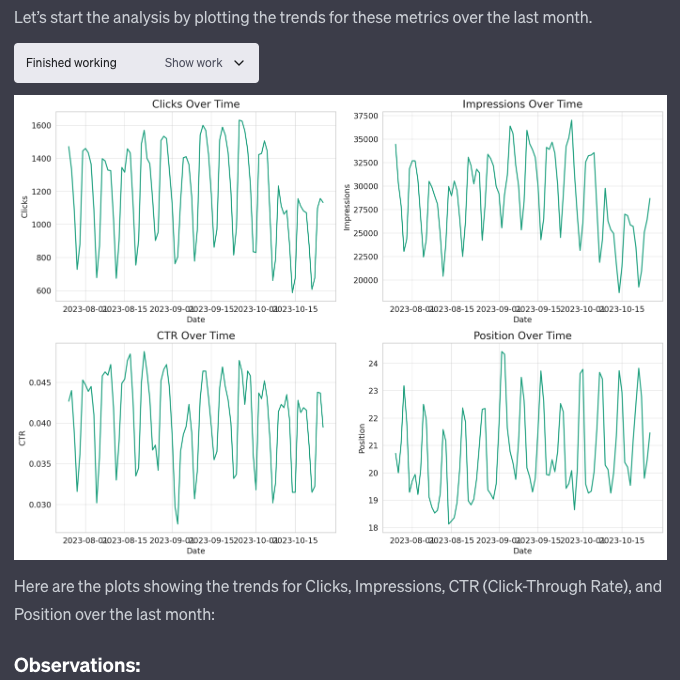
The jagged spikes got it confused, so I had to explain the peaks are from weekdays, and the valleys are from weekends. After this clarification, it calculated the dip and when it started. Like a good data analyst, it also proposed next steps to move the analysis forward:

And gave me solid tips on how to improve results:

And sent me downloadable files on what I should fix:
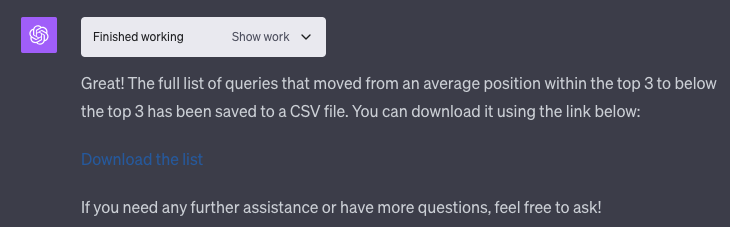
In the end, I learned a few things:
- Drop in clicks mirrored drop in impressions
- Average position did slip, but not enough to explain the drop
- This suggests the problem is mostly driven by a decline in search volume, which appears to be seasonal given last year’s data
- But even a small slip (e.g., falling out of the top 3 or first page) can trigger outsized drops in impressions, so I also have a list of pages I need to fix
In a way, data analysis with ChatGPT feels like the equivalent of a modern-day calculator. Why analyze by hand when you can have a machine execute flawlessly?
Sure, it struggled with amnesia. Sure, it can try to force an answer when it doesn’t have the data yet. But if you have a grasp of how the work should be done, you no longer have to do it alone.
Secrets are changing
I’ve worked with Excel wizards. Some people can churn out the fanciest models, but not be able to explain the point of the model. Knowing how to execute was their “secret sauce”. The value pendulum seems to be shifting away from this.
There are still legacy systems that only a few wizards can conjure magic in. But for anything modern and ubiquitous, we can lean on technical chatbots.
Whether it’s coding a subscription product, simple interactive website, or doing data deep-dives, it helps to understand what's involved, so you know how to prompt the chatbot and get it unstuck. But mastering the micro-quirks of a technical language isn’t the skill monopoly it used to be. Executing the minutiae is turning into a commodity.
Understanding the larger goal of a project, and how to differentiate it among a sea of same-ness is, increasingly, the whole point.
Speaking of, many of the AI businesses that have exploded in popularity will probably suffer a severe blow in the coming months. I’m more bullish on OpenAI’s GPT model, which makes me bearish on glorified frontend wrappers — pretty packages built off the GPT engine.
Chatting with a PDF, writing spreadsheet formulas started as great point solutions, but unless they can embed tightly into the customer’s workflow, ChatGPT's advances will eat their future growth.
The GPT wrappers that continue to thrive will need secrets of their own — while the world sees a commodity feature, they’re building a tight integration understood and valued by a select group of customers.
Here’s an example: the analysis I ran earlier works great with ChatGPT, but if I could automate this, get a monthly report, or even better, code suggestions and ideas to boost ranking, that’s worth an extra paid subscription.
SEO software like Ahrefs charges an arm and a leg for data tracking, but actionable insights are worth infinitely more. And if it can draft improvements that I only need to review before shipping, that is the real magic.
My bet is that covering this last mile of the user experience is crucial for differentiation. ChatGPT aims to be the gold-standard for digital intelligence, so GPT apps need to go deep into the customer’s world to stay mission-critical.
Do what the market leader has no bandwidth or incentive to do. When they care about breadth, there’s value in more depth.